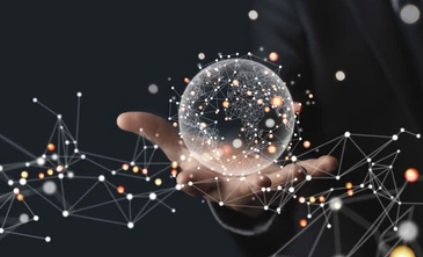
SecurityRisksinVision-BasedBeamPrediction:FromSpatialProxyAttackstoFeatureRefinement
The rapid evolution towards the sixth-generation (6G) networks demands advanced beamforming techniques to address challenges in dynamic, high-mobility scenarios, such as vehicular communications. Vision-based beam prediction utilizing RGB camera images emerges as a promising solution for accurate and responsive beam selection. However, reliance on visual data introduces unique vulnerabilities, particularly susceptibility to adversarial attacks, thus potentially compromising beam accuracy and overall network reliability. In this paper, we conduct the first systematic exploration of adversarial threats specifically targeting vision-based mmWave beam selection systems. Traditional white-box attacks are impractical in this context because ground-truth beam indices are inaccessible and spatial dynamics are complex. To address this, we propose a novel black-box adversarial attack strategy, termed Spatial Proxy Attack (SPA), which leverages spatial correlations between user positions and beam indices to craft effective perturbations without requiring access to model parameters or labels. To counteract these adversarial vulnerabilities, we formulate an optimization framework aimed at simultaneously enhancing beam selection accuracy under clean conditions and robustness against adversarial perturbations. We introduce a hybrid deep learning architecture integrated with a dedicated Feature Refinement Module (FRM), designed to systematically filter irrelevant, noisy and adversarially perturbed visual features. Evaluations using standard backbone models such as ResNet-50 and MobileNetV2 demonstrate that our proposed method significantly improves performance, achieving up to an +21.07\% gain in Top-K accuracy under clean conditions and a 41.31\% increase in Top-1 adversarial robustness compared to different baseline models.
相关推荐
热门文章
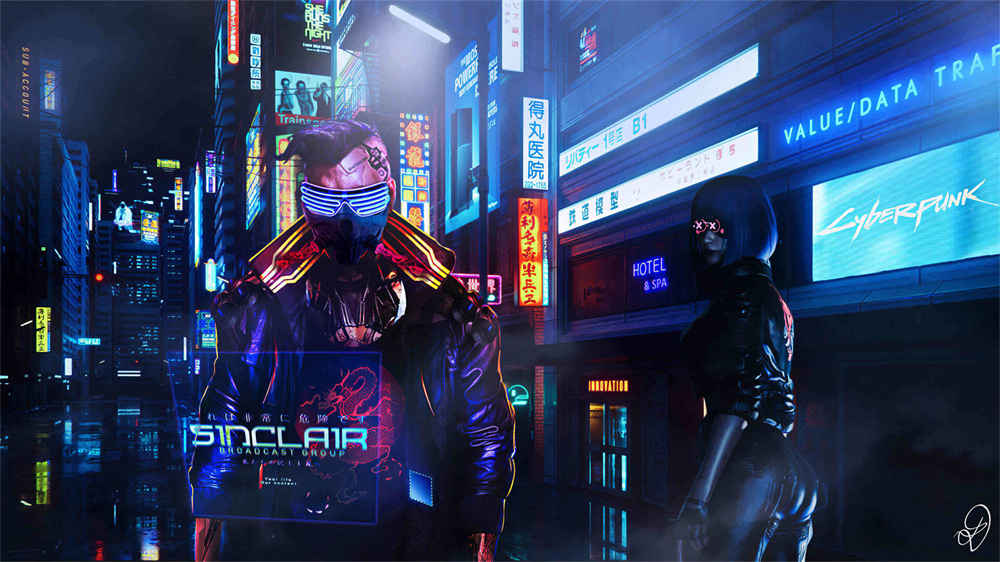