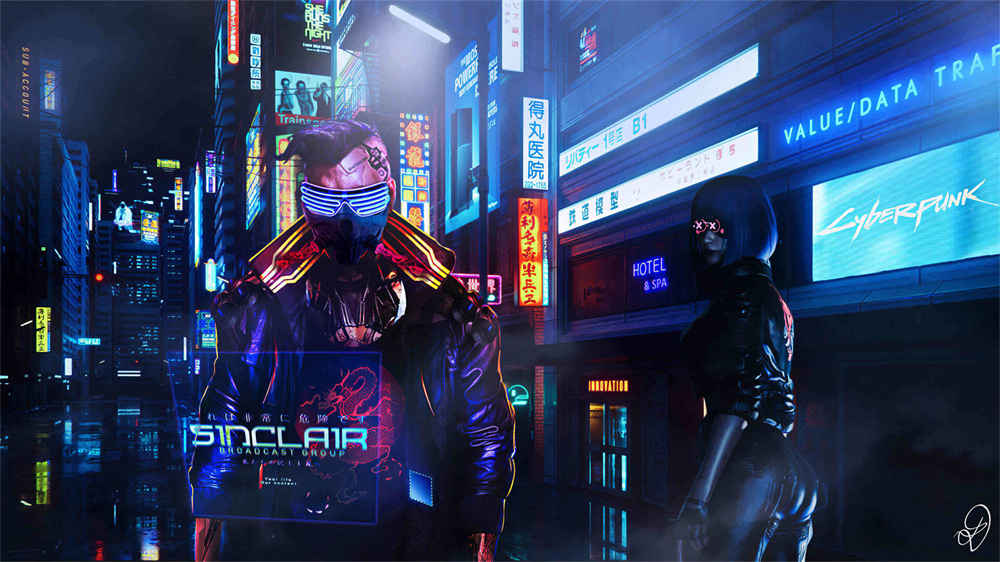
YourImageGeneratorIsYourNewPrivateDataset
Generative diffusion models have emerged as powerful tools to synthetically produce training data, offering potential solutions to data scarcity and reducing labelling costs for downstream supervised deep learning applications. However, effectively leveraging text-conditioned image generation for building classifier training sets requires addressing key issues: constructing informative textual prompts, adapting generative models to specific domains, and ensuring robust performance. This paper proposes the Text-Conditioned Knowledge Recycling (TCKR) pipeline to tackle these challenges. TCKR combines dynamic image captioning, parameter-efficient diffusion model fine-tuning, and Generative Knowledge Distillation techniques to create synthetic datasets tailored for image classification. The pipeline is rigorously evaluated on ten diverse image classification benchmarks. The results demonstrate that models trained solely on TCKR-generated data achieve classification accuracies on par with (and in several cases exceeding) models trained on real images. Furthermore, the evaluation reveals that these synthetic-data-trained models exhibit substantially enhanced privacy characteristics: their vulnerability to Membership Inference Attacks is significantly reduced, with the membership inference AUC lowered by 5.49 points on average compared to using real training data, demonstrating a substantial improvement in the performance-privacy trade-off. These findings indicate that high-fidelity synthetic data can effectively replace real data for training classifiers, yielding strong performance whilst simultaneously providing improved privacy protection as a valuable emergent property. The code and trained models are available in the accompanying open-source repository.
相关推荐
热门文章
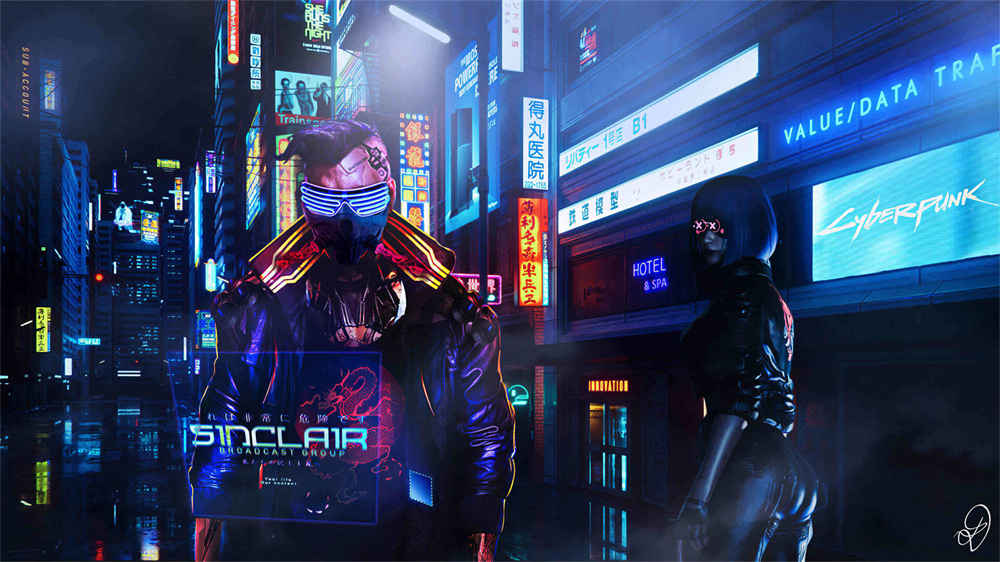