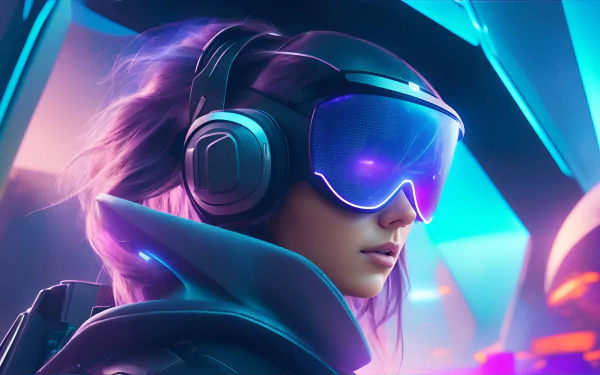
EnseSmells:Deepensembleandprogramminglanguagemodelsforautomatedcodesmellsdetection
A smell in software source code denotes an indication of suboptimal design and implementation decisions, potentially hindering the code understanding and, in turn, raising the likelihood of being prone to changes and faults. Identifying these code issues at an early stage in the software development process can mitigate these problems and enhance the overall quality of the software. Current research primarily focuses on the utilization of deep learning-based models to investigate the contextual information concealed within source code instructions to detect code smells, with limited attention given to the importance of structural and design-related features. This paper proposes a novel approach to code smell detection, constructing a deep learning architecture that places importance on the fusion of structural features and statistical semantics derived from pre-trained models for programming languages. We further provide a thorough analysis of how different source code embedding models affect the detection performance with respect to different code smell types. Using four widely-used code smells from well-designed datasets, our empirical study shows that incorporating design-related features significantly improves detection accuracy, outperforming state-of-the-art methods on the MLCQ dataset with with improvements ranging from 5.98% to 28.26%, depending on the type of code smell.
相关推荐
热门文章
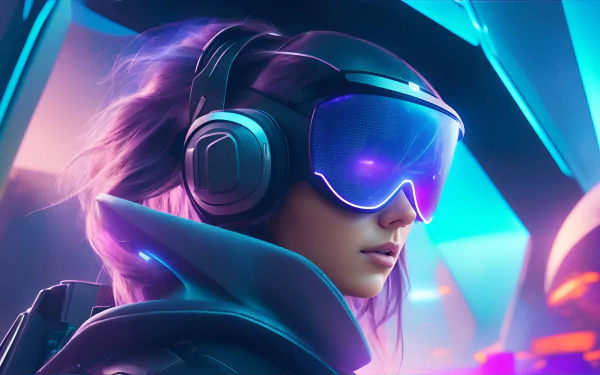