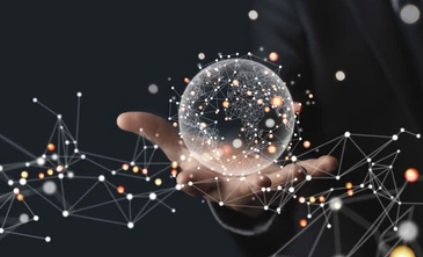
CombiningCausalModelsforMoreAccurateAbstractionsofNeuralNetworks
Mechanistic interpretability aims to reverse engineer neural networks by uncovering which high-level algorithms they implement. Causal abstraction provides a precise notion of when a network implements an algorithm, i.e., a causal model of the network contains low-level features that realize the high-level variables in a causal model of the algorithm. A typical problem in practical settings is that the algorithm is not an entirely faithful abstraction of the network, meaning it only partially captures the true reasoning process of a model. We propose a solution where we combine different simple high-level models to produce a more faithful representation of the network. Through learning this combination, we can model neural networks as being in different computational states depending on the input provided, which we show is more accurate to GPT 2-small fine-tuned on two toy tasks. We observe a trade-off between the strength of an interpretability hypothesis, which we define in terms of the number of inputs explained by the high-level models, and its faithfulness, which we define as the interchange intervention accuracy. Our method allows us to modulate between the two, providing the most accurate combination of models that describe the behavior of a neural network given a faithfulness level.
相关推荐
热门文章
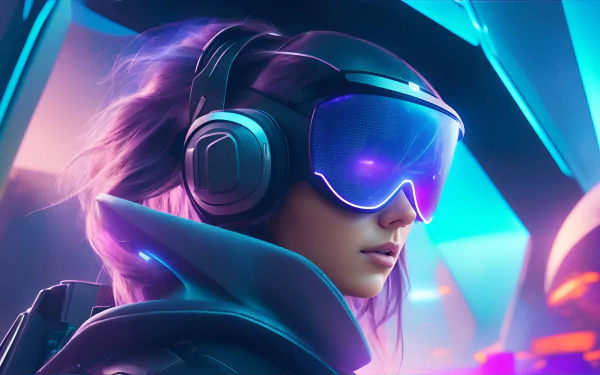