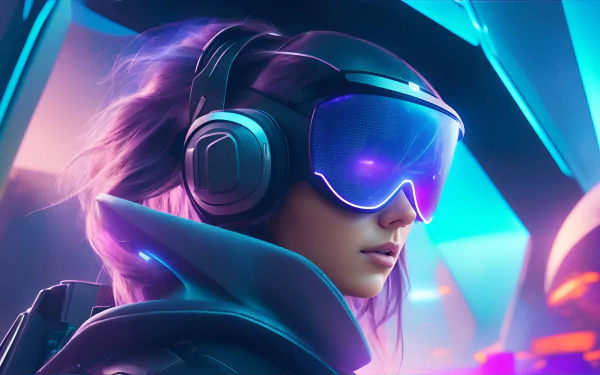
IterativeMulti-AgentReinforcementLearning:ANovelApproachTowardReal-WorldMulti-EchelonInventoryOptimization
Multi-echelon inventory optimization (MEIO) is critical for effective supply chain management, but its inherent complexity can pose significant challenges. Heuristics are commonly used to address this complexity, yet they often face limitations in scope and scalability. Recent research has found deep reinforcement learning (DRL) to be a promising alternative to traditional heuristics, offering greater versatility by utilizing dynamic decision-making capabilities. However, since DRL is known to struggle with the curse of dimensionality, its relevance to complex real-life supply chain scenarios is still to be determined. This thesis investigates DRL's applicability to MEIO problems of increasing complexity. A state-of-the-art DRL model was replicated, enhanced, and tested across 13 supply chain scenarios, combining diverse network structures and parameters. To address DRL's challenges with dimensionality, additional models leveraging graph neural networks (GNNs) and multi-agent reinforcement learning (MARL) were developed, culminating in the novel iterative multi-agent reinforcement learning (IMARL) approach. IMARL demonstrated superior scalability, effectiveness, and reliability in optimizing inventory policies, consistently outperforming benchmarks. These findings confirm the potential of DRL, particularly IMARL, to address real-world supply chain challenges and call for additional research to further expand its applicability.
相关推荐
热门文章
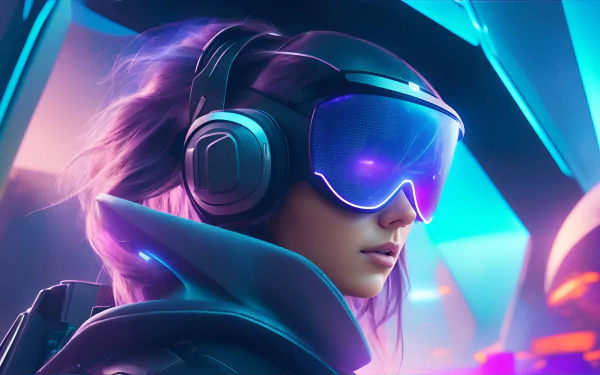