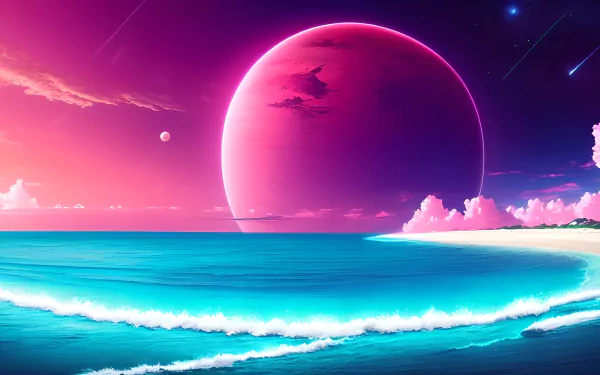
ConvergentNMPC-basedReinforcementLearningUsingDeepExpectedSarsaandNonlinearTemporalDifferenceLearning
In this paper, we present a learning-based nonlinear model predictive controller (NMPC) using an original reinforcement learning (RL) method to learn the optimal weights of the NMPC scheme. The controller is used as the current action-value function of a deep Expected Sarsa where the subsequent action-value function, usually obtained with a secondary NMPC, is approximated with a neural network (NN). With respect to existing methods, we add to the NN's input the current value of the NMPC's learned parameters so that the network is able to approximate the action-value function and stabilize the learning performance. Additionally, with the use of the NN, the real-time computational burden is approximately halved without affecting the closed-loop performance. Furthermore, we combine gradient temporal difference methods with parametrized NMPC as function approximator of the Expected Sarsa RL method to overcome the potential parameters divergence and instability issues when nonlinearities are present in the function approximation. The simulation result shows that the proposed approach converges to a locally optimal solution without instability problems.
相关推荐
热门文章
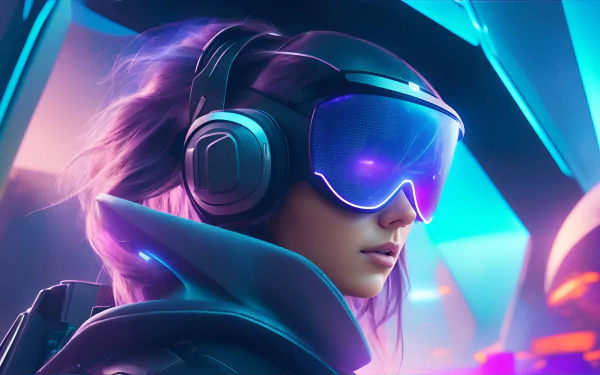