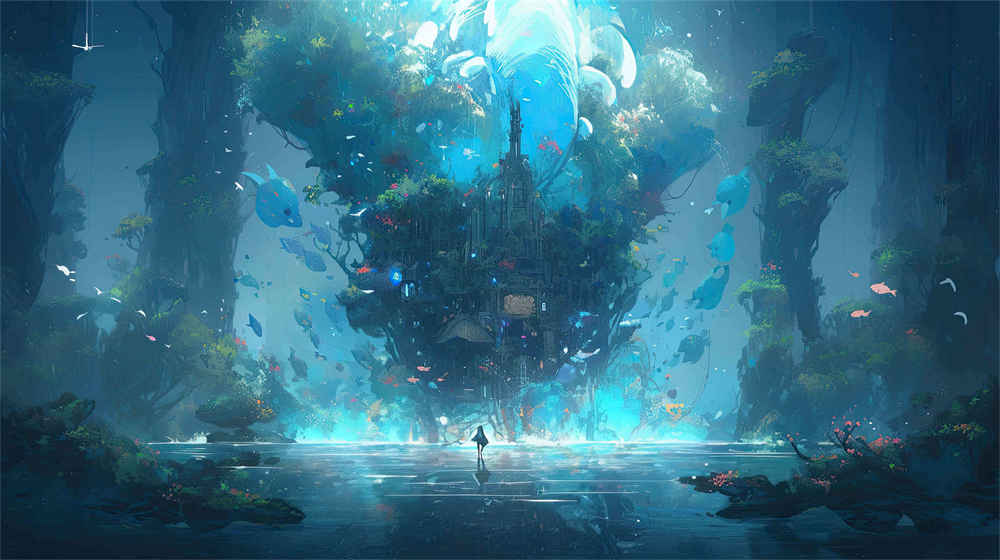
SyntheticSpectroscopyforWhiteDwarfClassification:AddressingLabelUncertaintyandClassImbalance
With the imminent data releases from next-generation spectroscopic surveys, hundreds of thousands of white dwarf spectra are expected to become available within the next few years, increasing the data volume by an order of magnitude. This surge in data has created a pressing need for automated tools to efficiently analyze and classify these spectra. Although machine learning algorithms have recently been applied to classify large spectroscopic datasets, they remain constrained by the limited availability of training data. The Sloan Digital Sky Survey (SDSS) serves as the current standard training set, as it provides the largest collection of labeled spectra; however, it faces challenges related to severe class imbalance and uncertain label consistency across different surveys. In this work, we address these limitations by training histogram gradient-boosted classifiers on a synthetic SDSS dataset to identify six ubiquitous chemical signatures in the atmosphere of white dwarfs, and test them on 14,246 objects with SNR$>$10 SDSS spectroscopy. We show our approach not only surpasses human expert performance, but also enables subtype classification and effectively resolves label transferability issues. The methodology developed here is adaptable to any spectroscopic survey, providing a critical tool for the astronomical community.
相关推荐
热门文章
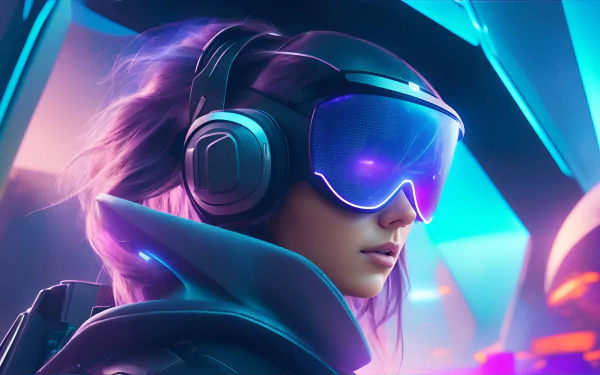