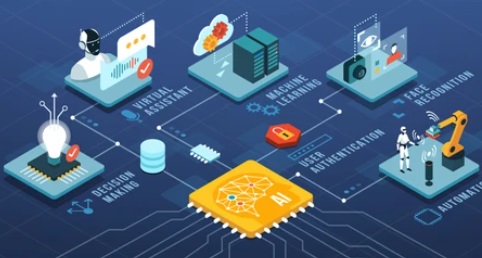
SpecReX:ExplainableAIforRamanSpectroscopy
Raman spectroscopy is becoming more common for medical diagnostics with deep learning models being increasingly used to leverage its full potential. However, the opaque nature of such models and the sensitivity of medical diagnosis together with regulatory requirements necessitate the need for explainable AI tools. We introduce SpecReX, specifically adapted to explaining Raman spectra. SpecReX uses the theory of actual causality to rank causal responsibility in a spectrum, quantified by iteratively refining mutated versions of the spectrum and testing if it retains the original classification. The explanations provided by SpecReX take the form of a responsibility map, highlighting spectral regions most responsible for the model to make a correct classification. To assess the validity of SpecReX, we create increasingly complex simulated spectra, in which a "ground truth" signal is seeded, to train a classifier. We then obtain SpecReX explanations and compare the results with another explainability tool. By using simulated spectra we establish that SpecReX localizes to the known differences between classes, under a number of conditions. This provides a foundation on which we can find the spectral features which differentiate disease classes. This is an important first step in proving the validity of SpecReX.
相关推荐
热门文章
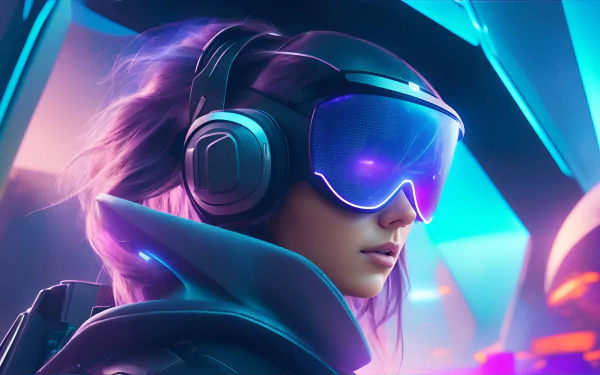