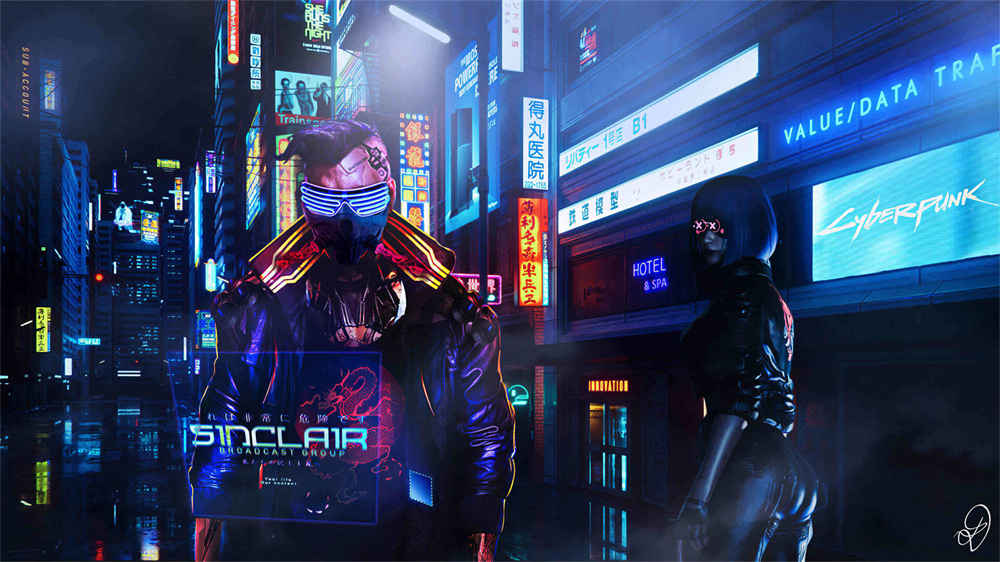
AutomatedCellStructureExtractionfor3DElectronMicroscopybyDeepLearning
Modeling the 3D structures of cells and tissues is crucial in biology. Sequential cross-sectional images from electron microscopy provide high-resolution intracellular structure information. Segmentation of complex cell structures remains a laborious manual task for experts, demanding time and effort. This bottleneck in analyzing biological images requires efficient and automated solutions. This study explores deep learning-based automated segmentation of biological images, enabling accurate reconstruction of the 3D structures of cells and organelles. We constructed an analysis system for the cell images of Cyanidioschyzon merolae, a primitive unicellular red algae. This system utilizes sequential cross-sectional images captured by Focused Ion Beam Scanning Electron Microscopes (FIB-SEM). We adopted the U-Net and performed pre-training to identify and segment cell organelles from single-cell images. In addition, we employed the Segmentation Anything Model (SAM) and the 3D watershed algorithm to extract individual 3D images of each cell from large-scale microscope images containing numerous cells. Finally, we applied the pre-trained U-Net to segment each structure within these 3D images. Through this procedure, we could fully automate the creation of 3D cell models. Our approach would apply to other cell types, and we aim to build a versatile analysis system. We will also explore adopting other deep learning techniques and combinations of image processing methods to further enhance segmentation accuracy.
相关推荐
热门文章
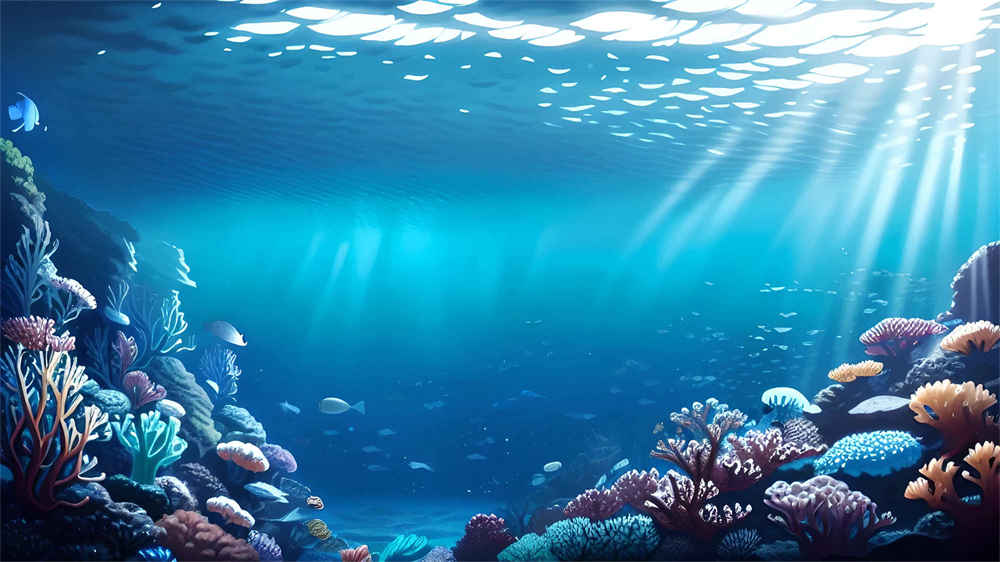