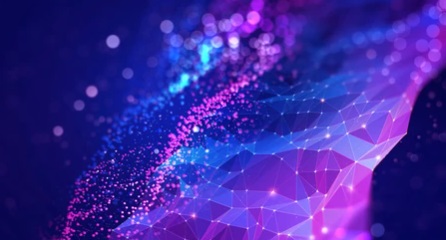
Co-DevelopingCausalGraphswithDomainExpertsGuidedbyWeightedFDR-Adjustedp-values
This paper proposes an approach facilitating co-design of causal graphs between subject matter experts and statistical modellers. Modern causal analysis starting with formulation of causal graphs provides benefits for robust analysis and well-grounded decision support. Moreover, this process can enrich the discovery and planning phase of data science projects.The key premise is that plotting relevant statistical information on a causal graph structure can facilitate an intuitive discussion between domain experts and modellers. Furthermore, Hand-crafting causality graphs, integrating human expertise with robust statistical methodology, enables ensuring responsible AI practices.The paper focuses on using multiplicity-adjusted p-values, controlling for the false discovery rate (FDR), as an aid for co-designing the graph. A family of hypotheses relevant to causal graph construction is identified, including assessing correlation strengths, directions of causal effects, and how well an estimated structural causal model induces the observed covariance structure.An iterative flow is described where an initial causal graph is drafted based on expert beliefs about likely causal relationships. The subject matter expert's beliefs, communicated as ranked scores could be incorporated into the control of the measure proposed by Benjamini and Kling, the FDCR (False Discovery Cost Rate). The FDCR-adjusted p-values then provide feedback on which parts of the graph are supported or contradicted by the data. This co-design process continues, adding, removing, or revising arcs in the graph, until the expert and modeller converge on a satisfactory causal structure grounded in both domain knowledge and data evidence.
相关推荐