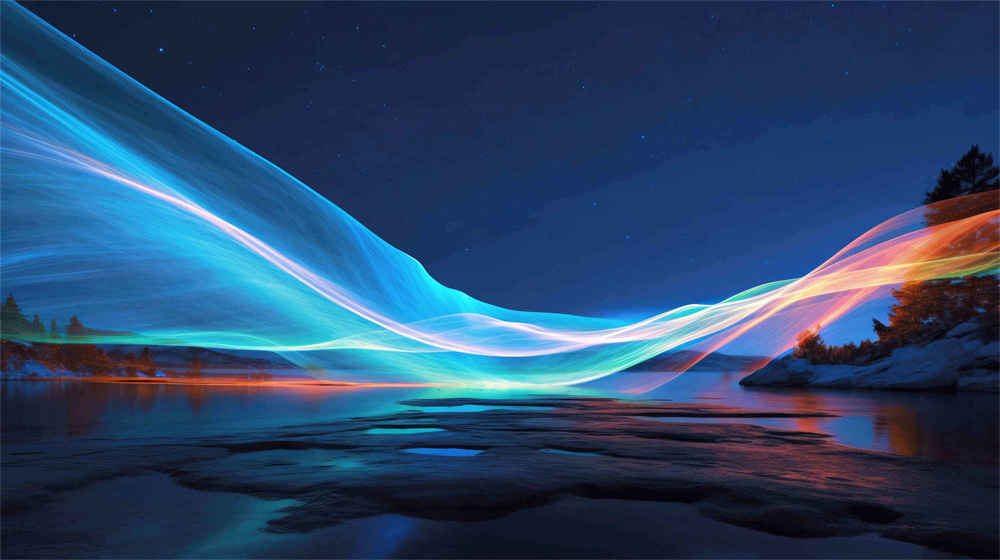
BetterBench:AssessingAIBenchmarks,UncoveringIssues,andEstablishingBestPractices
AI models are increasingly prevalent in high-stakes environments, necessitating thorough assessment of their capabilities and risks. Benchmarks are popular for measuring these attributes and for comparing model performance, tracking progress, and identifying weaknesses in foundation and non-foundation models. They can inform model selection for downstream tasks and influence policy initiatives. However, not all benchmarks are the same: their quality depends on their design and usability. In this paper, we develop an assessment framework considering 46 best practices across an AI benchmark's lifecycle and evaluate 24 AI benchmarks against it. We find that there exist large quality differences and that commonly used benchmarks suffer from significant issues. We further find that most benchmarks do not report statistical significance of their results nor allow for their results to be easily replicated. To support benchmark developers in aligning with best practices, we provide a checklist for minimum quality assurance based on our assessment. We also develop a living repository of benchmark assessments to support benchmark comparability, accessible at this http URL.
相关推荐