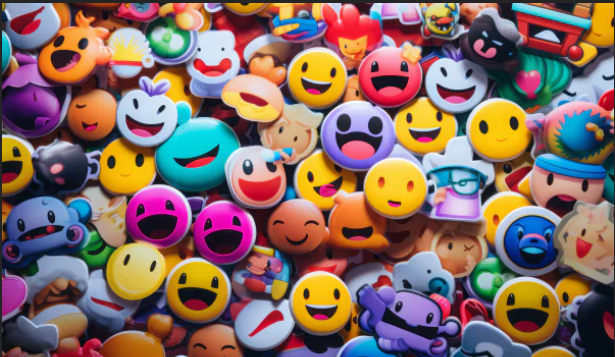
Unleashingthepowerofnovelconditionalgenerativeapproachesfornewmaterialsdiscovery
For a very long time, computational approaches to the design of new materials have relied on an iterative process of finding a candidate material and modeling its properties. AI has played a crucial role in this regard, helping to accelerate the discovery and optimization of crystal properties and structures through advanced computational methodologies and data-driven approaches. To address the problem of new materials design and fasten the process of new materials search, we have applied latest generative approaches to the problem of crystal structure design, trying to solve the inverse problem: by given properties generate a structure that satisfies them without utilizing supercomputer powers. In our work we propose two approaches: 1) conditional structure modification: optimization of the stability of an arbitrary atomic configuration, using the energy difference between the most energetically favorable structure and all its less stable polymorphs and 2) conditional structure generation. We used a representation for materials that includes the following information: lattice, atom coordinates, atom types, chemical features, space group and formation energy of the structure. The loss function was optimized to take into account the periodic boundary conditions of crystal structures. We have applied Diffusion models approach, Flow matching, usual Autoencoder (AE) and compared the results of the models and approaches. As a metric for the study, physical PyMatGen matcher was employed: we compare target structure with generated one using default tolerances. So far, our modifier and generator produce structures with needed properties with accuracy 41% and 82% respectively. To prove the offered methodology efficiency, inference have been carried out, resulting in several potentially new structures with formation energy below the AFLOW-derived convex hulls.
相关推荐
热门文章
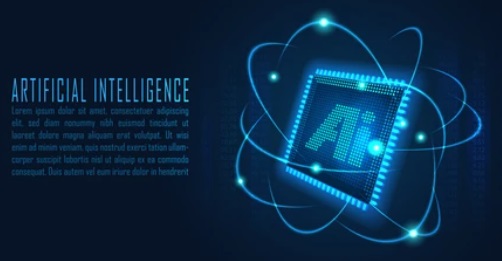