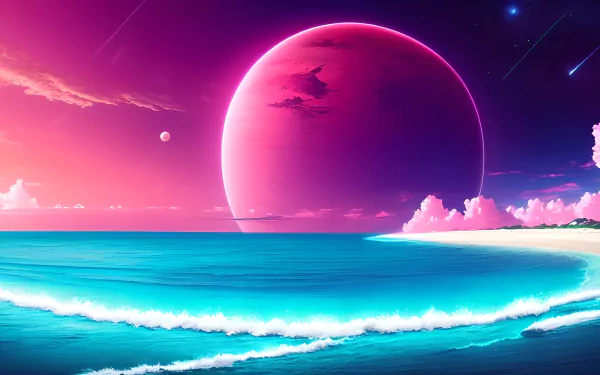
MachineLearning-BasedEnhancementsofEmpiricalEnergyFunctions:Structure,DynamicsandSpectroscopyofModifiedBenzenes
The effect of replacing individual contributions to an empirical energy function are assessed for halogenated benzenes (X-Bz, X = H, F, Cl, Br) and chlorinated phenols (Cl-PhOH). Introducing electrostatic models based on distributed charges (MDCM) instead of usual atom-centered point charges yields overestimated hydration free energies unless the van der Waals parameters are reparametrized. Scaling van der Waals ranges by 10 \% to 20 \% for three Cl-PhOH and most X-Bz yield results within experimental error bars, which is encouraging, whereas for benzene (H-Bz) point charge-based models are sufficient. Replacing the bonded terms by a neural network-trained energy function with either fluctuating charges or MDCM electrostatics also yields qualitatively correct hydration free energies which still require adaptation of the van der Waals parameters. The infrared spectroscopy of Cl-PhOH is rather well predicted by all models although the ML-based energy function performs somewhat better in the region of the framework modes. It is concluded that refinements of empirical energy functions for targeted applications is a meaningful way towards more quantitative simulations.
相关推荐