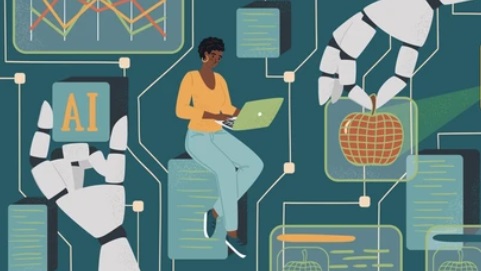
SiMilarity-EnhancedHomophilyforMulti-ViewHeterophilousGraphClustering
With the increasing prevalence of graph-structured data, multi-view graph clustering has been widely used in various downstream applications. Existing approaches primarily rely on a unified message passing mechanism, which significantly enhances clustering performance. Nevertheless, this mechanism limits its applicability to heterophilous situations, as it is fundamentally predicated on the assumption of homophily, i.e., the connected nodes often belong to the same class. In reality, this assumption does not always hold; a moderately or even mildly homophilous graph is more common than a fully homophilous one due to inevitable heterophilous information in the graph. To address this issue, in this paper, we propose a novel SiMilarity-enhanced Homophily for Multi-view Heterophilous Graph Clustering (SMHGC) approach. By analyzing the relationship between similarity and graph homophily, we propose to enhance the homophily by introducing three similarity terms, i.e., neighbor pattern similarity, node feature similarity, and multi-view global similarity, in a label-free manner. Then, a consensus-based inter- and intra-view fusion paradigm is proposed to fuse the improved homophilous graph from different views and utilize them for clustering. The state-of-the-art experimental results on both multi-view heterophilous and homophilous datasets collectively demonstrate the strong capacity of similarity for unsupervised multi-view heterophilous graph learning. Additionally, the consistent performance across semi-synthetic datasets with varying levels of homophily serves as further evidence of SMHGC's resilience to heterophily.
相关推荐