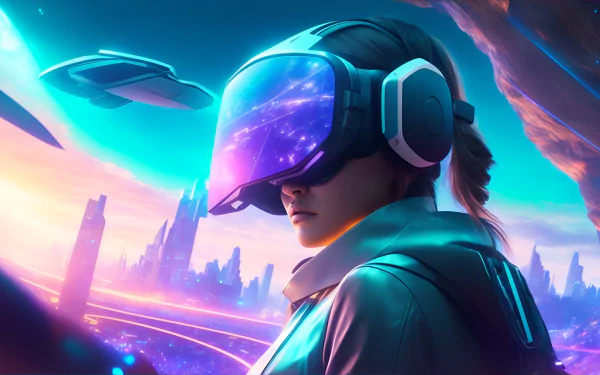
DifferentialPrivacyRegularization:ProtectingTrainingDataThroughLossFunctionRegularization
Training machine learning models based on neural networks requires large datasets, which may contain sensitive information. The models, however, should not expose private information from these datasets. Differentially private SGD [DP-SGD] requires the modification of the standard stochastic gradient descent [SGD] algorithm for training new models. In this short paper, a novel regularization strategy is proposed to achieve the same goal in a more efficient manner.
相关推荐
热门文章
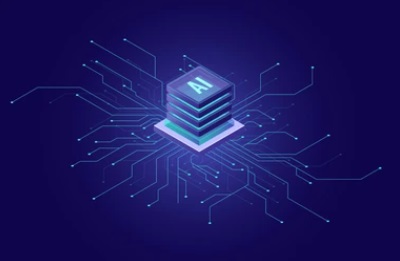