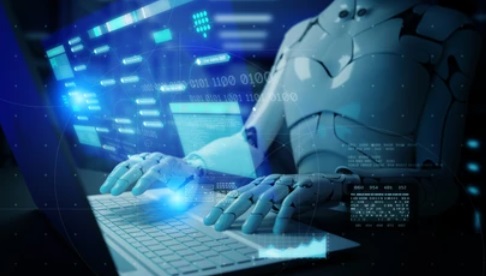
ReplacingPathswithConnection-BiasedAttentionforKnowledgeGraphCompletion
Knowledge graph (KG) completion aims to identify additional facts that can be inferred from the existing facts in the KG. Recent developments in this field have explored this task in the inductive setting, where at test time one sees entities that were not present during training; the most performant models in the inductive setting have employed path encoding modules in addition to standard subgraph encoding modules. This work similarly focuses on KG completion in the inductive setting, without the explicit use of path encodings, which can be time-consuming and introduces several hyperparameters that require costly hyperparameter optimization. Our approach uses a Transformer-based subgraph encoding module only; we introduce connection-biased attention and entity role embeddings into the subgraph encoding module to eliminate the need for an expensive and time-consuming path encoding module. Evaluations on standard inductive KG completion benchmark datasets demonstrate that our Connection-Biased Link Prediction (CBLiP) model has superior performance to models that do not use path information. Compared to models that utilize path information, CBLiP shows competitive or superior performance while being faster. Additionally, to show that the effectiveness of connection-biased attention and entity role embeddings also holds in the transductive setting, we compare CBLiP's performance on the relation prediction task in the transductive setting.
相关推荐
热门文章
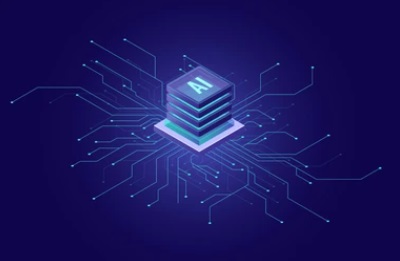