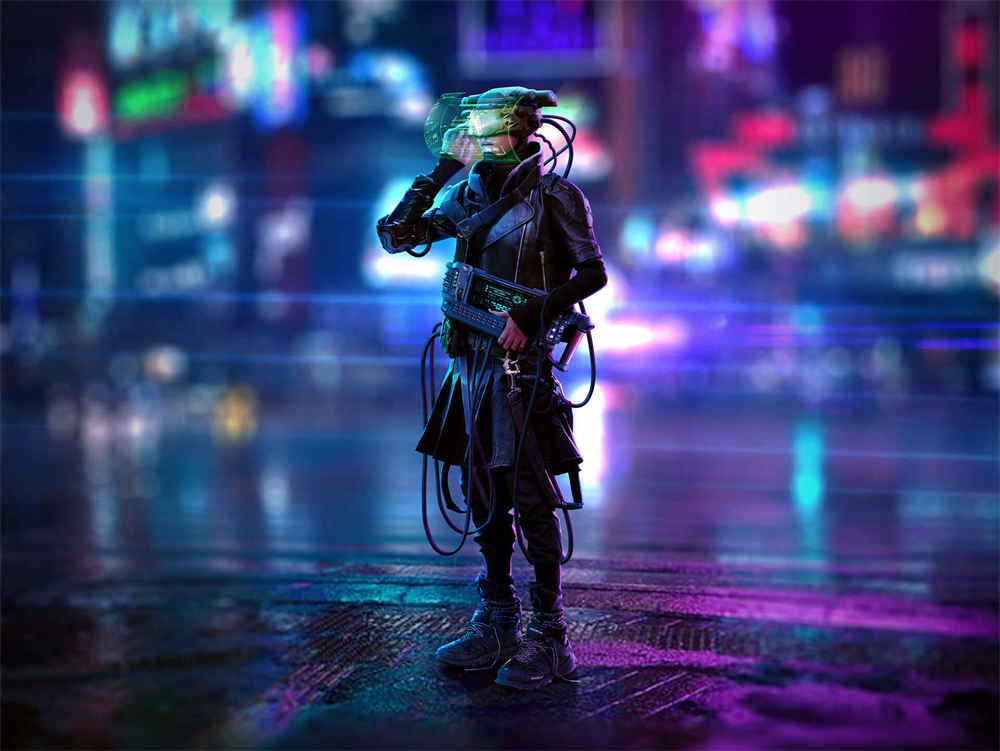
QuantumStateReconstructioninaNoisyEnvironmentviaDeepLearning
Quantum noise is currently limiting efficient quantum information processing and computation. In this work, we consider the tasks of reconstructing and classifying quantum states corrupted by the action of an unknown noisy channel using classical feedforward neural networks. By framing reconstruction as a regression problem, we show how such an approach can be used to recover with fidelities exceeding 99% the noiseless density matrices of quantum states of up to three qubits undergoing noisy evolution, and we test its performance with both single-qubit (bit-flip, phase-flip, depolarising, and amplitude damping) and two-qubit quantum channels (correlated amplitude damping). Moreover, we also consider the task of distinguishing between different quantum noisy channels, and show how a neural network-based classifier is able to solve such a classification problem with perfect accuracy.
相关推荐