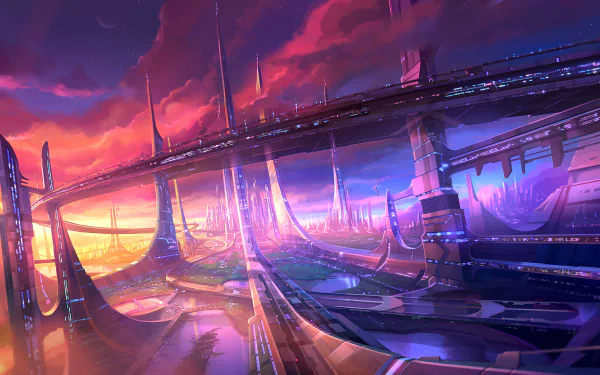
Deepgenerativemodelconditionedbyphasepicksforsynthesizinglabeledseismicwaveformswithlimiteddata
Shortage of labeled seismic field data poses a significant challenge for deep-learning related applications in seismology. One approach to mitigate this issue is to use synthetic waveforms as a complement to field data. However, traditional physics-driven methods for synthesizing data are computationally expensive and often fail to capture complex features in real seismic waveforms. In this study, we develop a deep-learning-based generative model, PhaseGen, for synthesizing realistic seismic waveforms dictated by provided P- and S-wave arrival labels. Contrary to previous generative models which require a large amount of data for training, the proposed model can be trained with only 100 seismic events recorded by a single seismic station. The fidelity, diversity and alignment for waveforms synthesized by PhaseGen with diverse P- and S-wave arrival labels are quantitatively evaluated. Also, PhaseGen is used to augment a labelled seismic dataset used for training a deep neural network for the phase picking task, and it is found that the picking capability trained with the augmented dataset is unambiguously improved. It is expected that PhaseGen can offer a valuable alternative for synthesizing realistic waveforms and provide a promising solution for the lack of labeled seismic data.
相关推荐